Past Research Areas:
CellProfiler and other bioimage analysis software
We launched and led the open-source CellProfiler project for 18 years. It is beloved by tens of thousands of biologists around the world. Since 2021, the Cimini lab is leading development (CellProfiler site).
CellProfiler Analyst: Machine learning for high-content screens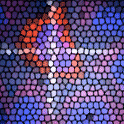
We launched and led the open-source CellProfiler Analyst project for 16 years. It helps biologists to train supervised machine learning algorithms to identify complex phenotype in high-throughput microscopy experiments. Since 2021, the Cimini lab is leading development (CellProfiler Analyst site).
Quantifying dynamic phenotypes
Many biological questions can only be investigated by collecting time-lapse movies. We have analyzed these images to identify, for example, novel cell cycle landmarks and motor protein regulators. We have also integrated this data with flow cytometry data to quantify unusual cell cycle outcomes.
Imaging flow cytometry
Imaging flow cytometry combines the high-throughput nature of flow cytometry with the high-resolution nature of fluorescence microscopy. For each experimental sample, it yields hundreds of thousands of images of individual cells. We are developing methods to mine these large datasets [NSF project page].
Co-culture systems
In co-cultured cell systems, two or more cell types are grown together in order to maintain more native physiological functions, enabling experiments that test genetic and chemical perturbations in a more realistic environment. We have developed image analysis approaches to extract information from fluorescence microscopy images of these cell systems, enabling experiments in liver regeneration and hepatotoxicity [NSF CAREER project page].
Quantifying C. elegans
The worm C. elegans can be robotically prepared and imaged and is an effective model to probe a variety of biological questions that require whole animals rather than isolated cells. We have developed sorely needed C. elegans analysis algorithms and validated them in specific large-scale experiments to identify regulators of fat metabolism and pathogen infection.